http://www.defenseone.com/technology/2015/04/can-military-make-prediction-machine/109561/
U.S. Navy photo by Mass Communication Specialist 3rd Class John Fischer
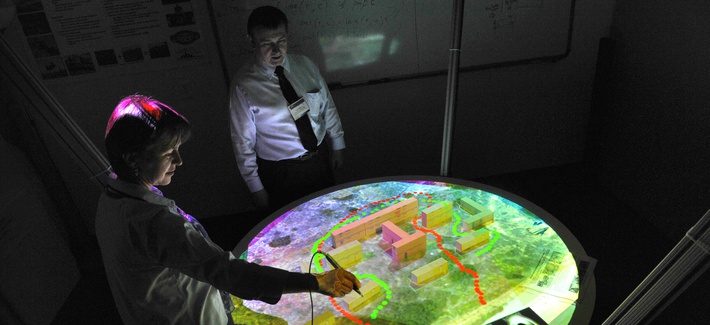
Can the Military Make a Prediction Machine?
The planet is awash in open, free data. Can military-funded research turn it into a crystal ball?
What could the military do if it could
better understand the massive amounts of data that humanity creates, an
estimated 2.5 quintillion bytes every day? Could it predict aspects of
the future?
If Pentagon funds can help create—even partially—a machine capable of understanding cause and effect, or causality, and do so on the scale of thousands of signals, data points, and possible conclusions, then, perhaps, big data will reach its real potential: a predictive tool that allows leaders to properly position soldiers, police forces, and humanitarian relief long before the action starts.
Among the military programs probing this new realm is Big Mechanism, run by the Defense Advanced Research Projects Agency, or DARPA. It seeks to turn machine-collected (or machine-generated) data into real insights into complex systems, and do so automatically.
Some, such as Wired’s Chris Anderson, have suggested that access to huge amounts of data, which makes correlational analysis easier, has made old-fashioned, theory-based science obsolete. But in a recent conversation with Defense One, DARPA program manager Paul Cohen said he was looking more to mechanize the human capacity for causation, rather than innovate around it. “We’re a very much aiming toward a new science, but we’re very much interested in causal relationships,” he said. “What we’re finding is that mathematical modeling of systems is very hard to maintain.”
The supply of data, it turns out, is growing too quickly for the human race to use it effectively to solve big problems. The expanding reach and power of computational intelligence is both cause and, at least potentially, cure.
“Having big data about complicated economic, biological, neural and climate systems isn’t the same as understanding the dense webs of causes and effects—what we call the big mechanisms—in these systems,” Cohen said last year. “Unfortunately, what we know about big mechanisms is contained in enormous, fragmentary and sometimes contradictory literatures and databases, so no single human can understand a really complicated system in its entirety. Computers must help us.”
These big systems can be as large as the entire world or as small as cancer cells, an initial area of focus for the program.
Machine intelligence can collect and process data on a scale unimaginable to regular humans. But processing data is very different from making sense of it, and from making predictions. If we could get computer systems to predict in the way that humans do, but with the data and processing power only available to massively interconnected systems, could we open up areas of the future to new inference? Cohen has suggested that the answer is yes.
“The beautiful thing about causal models is that they make predictions, so we can return to our big data and see whether we’re [retrospectively] right,” Cohen said. “And we can propose new experiments, suggest interventions and advance our knowledge more rapidly.”
The ways in which humans interact with government, with one another, with medical facilities, transit systems and brands, etc. can predict events of national security significance. They can indicate, for instance, if a deadly disease outbreak is taking hold in a small rural community or if civil unrest is on the rise.
One example of that is the Open Source Indicators Program, launched in 2011 by from the Intelligence Advanced Research Programs agency. Led by program manager Jason Matheny, Open Source Indicators funds projects to predict events of national security relevance by monitoring tens of thousands of blogs, RSS feeds, news reports, social network chatter from sites like Twitter and Facebook, and other open sources.
Very early on, program participants began to generate some surprising results. In 2012, Virginia Tech computer scientist Naren Ramakrishnan, working solely with signals culled from the open Internet, effectively predicted both Mexico’s Yo Soy 132 protest movement, sometimes called the Mexican Arab Spring, and the “Friendship Bridge” protests that riled parts of Brazil and Paraguay.
Around the same time, Georgetown University data scientist Kalev Leetaru used a database of millions of open-source indicators to correctly (but retroactively) predict the spot in Abbottabad, Pakistan, where Osama Bin Laden was found.
But for every instance where big data correctly predicted a big national-security event, critics can point to a big miss. Last year, for example, such indicators failed to predict the Ebola outbreak.
The military and national security communities have only begun to explore the potential of big data to solve these kinds of enormously complex problems. But before open-source signal hunting can reach its full potential, people like Cohen and Matheny need to answer some serious questions.
Among them, how to balance privacy concerns with national security objectives? Open-source intel is, by definition, freely available on the Internet. But when most people give their data away, they don’t imagine military technologists trying to extrapolate predictions from that data. As more people become concerned about how their data is used, especially by government actors, they’re changing the data that they make and release.
Last summer, Lt. Gen. Michael Flynn, then the director of the Defense Intelligence Agency, discussed how the military had “completely revamped” the way it collects intelligence around open-source data. He said that, as the military’s reliance on such data grows, online behavior is changing and adapting. When asked if he was concerned by that, he answered, “Yes.”
Another question: how do we get machine intelligence to discover causation and not just correlation? How do you teach a big-data machine to provide a fully true answer, not just an output? Answer: we may need to re-design, on a fundamental level, the way we communicate with machines.
Perhaps one of the greatest paradoxes of the modern age is that our method for interacting with machine intelligence remains relatively crude: typing, and more and more with our thumbs. That limits our collaboration with machines to specific tasks with strict parameters.
When given a chance to collaborate with a machine or with a human on a project of any complexity, we’ll press zero to talk to the human almost every time. That’s a problem in a national-security context: service members are being asked to interact with an ever-larger number of systems in life-and-death situations.
Another one of Cohen’s DARPA programs, announced in February, seeks to change that. The Communicating with Computers effort seeks to “bridge the language barrier” between humans and machines, Cohen remarked in a press release.
“Human-machine communication falls short of the human-human standard, where speakers and listeners consider such contextual aspects as what has been said already, the purposes of the communication, the best ways to express ideas, who they are speaking with, prevailing social conventions and the availability of other modes of expression such as gestures. And so computers that might otherwise contribute more significantly to solving problems in a range of areas, including national security, remain in relatively simplistic roles such as crunching large datasets and providing driving directions.”
No comments:
Post a Comment